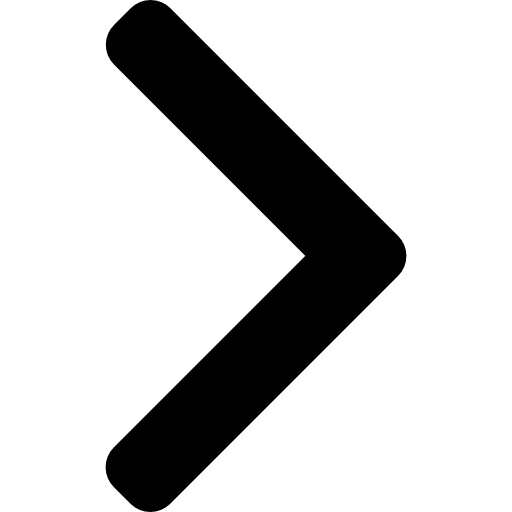
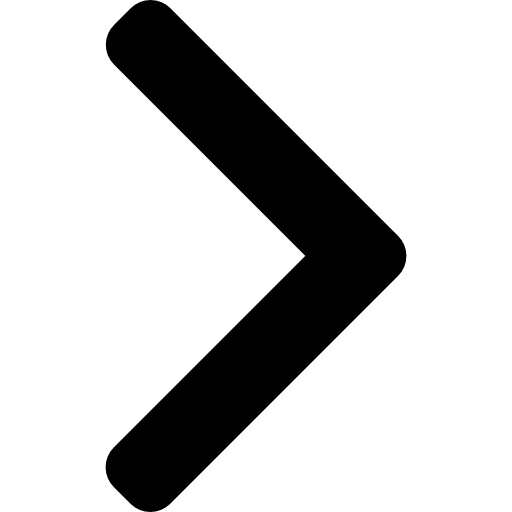
Organizations need the most recent Generative AI-powered tools to detect threats and protect their systems and data resources. By 2025, AI in Cybersecurity will upgrade the industry in multiple ways. Deep learning in cyber security can constantly boost the security process to recognize cyber risks and assist in shielding businesses from cyber-attacks. Artificial Intelligence in Cybersecurity such as AI-enabled Deep Learning processes will help enterprises maintain the security of their systems more efficiently and mitigate the risk of cyber threats.
To protect data, organizations need to constantly upgrade their cyber security system to fight all sorts of cybercrime and stay protected from cyberattacks. The Cybersecurity system should keep upgrading as malware and viruses are becoming more intelligent and evolving significantly. Robotics, Artificial intelligence (AI), and Deep Learning are altering the game's rules.
Any potential harmful attack that aims to gain unauthorized access to data, interference with digital activities, or contaminate information is a cyber security threat. Cyber threats may come from various sources, including corporate espionage, hacktivists, terrorist organizations, adversarial nation-states, criminal organizations, lone hackers, and disgruntled workers.
Security professionals fail at some point, even with new advancements in defense strategies. Current technologies sometimes jeopardize the organization's cyber security system. Combining the strength of Artificial Intelligence in Cybersecurity and the skills of security professionals, organizations can constantly monitor vulnerability checks and keep the system updated. Organizations benefit from instant insights, accurate threat predictions, and reduced response time.
The types of attacks we are currently vulnerable to are:
Sensitive data has been at risk due to cyberattacks in recent years. One hundred forty-three million people's personal information, including birth dates, addresses, and Social Security numbers, were leaked in the 2017 Equifax breach. Due to the frequent data breaches that plague high-profile companies, the world is experiencing a severe shortage of highly skilled cybersecurity workers. Due to the lack of proper cyber security systems, more and more cyber security threats are being reported consistently across the globe. Deep learning and Artificial Intelligence in Cybersecurity plays a vital role in analyzing the data, anticipating upcoming cyber threats, and upgrading the system to protect it from cyber threats.
Before we get into the benefits of AI in cybersecurity and how Deep Learning has become a part of the cybersecurity system, let's look at the most common threats that cybersecurity teams face today:
Deep learning exploits unknown elements in the input distribution throughout the training phase. While self-learning the cybersecurity system, deep learning algorithm relies on Artificial Neural Networks (ANN) that simulate how the brain processes information. Deep learning models use several algorithms to analyze data and prove the right threat predictions.
Deep Learning in cyber security employs scientific computing to deal with complicated issues. Deep learning processes in the cyber security system are more effective than other Generative AI methods for completing complex tasks. The deep learning algorithm is also well suited with a combination of various kinds of neural networks to handle large amounts of data, which is another advantage over other AI in cybersecurity methods.
Deep Learning in Cybersecurity is rapidly growing and evolving to be predictive. Cybersecurity is simultaneously becoming more affordable and equally effective. A deep learning algorithm creates patterns from a massive dataset to analyze the data and predict threats in real-time. Natural language processing (NLP), facial recognition, autonomous threat detection, and the development of automated security systems have all tremendously benefited from Generative AI and Deep Learning. Additionally, Deep learning in the cybersecurity system enables threat detection systems, foreseeing new cyber-attacks, and promptly notifying an admin of data breaches.
AI in cybersecurity and Deep learning can secure the cyber security system of any business and can protect them from cyber-attacks. It can identify and prevent attacks more quickly and accurately and help organizations better understand and protect their data. Over the past five years, Artificial Intelligence in Cybersecurity and deep learning has developed and is now widely used in various industries. In 2025, deep learning will be an indispensable part of the cybersecurity industry, and we will be at the forefront of this transformation. Visit our page for AI and Deep learning solutions to learn more.
You can subscribe to our blog to learn more about AI and Deep Learning in the cybersecurity world.