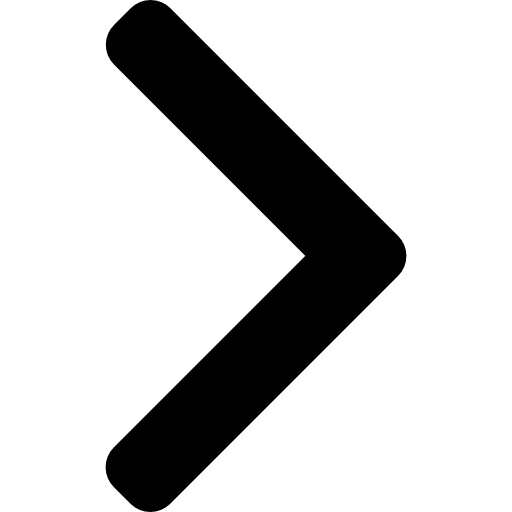
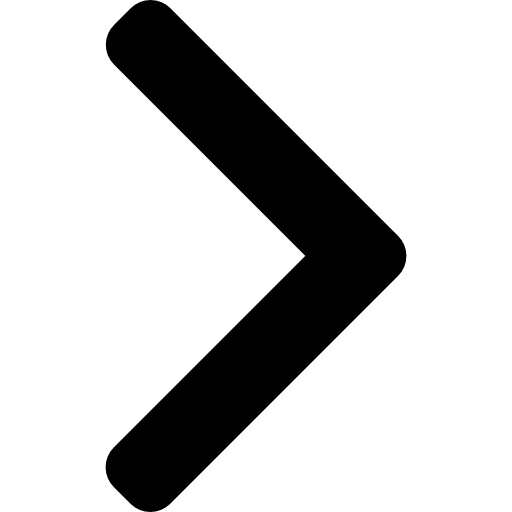
Protein is an essential macronutrient. Protein serves various cell metabolism, industrial catalysts, and medicines. Recent technological developments like AI in protein engineering have made it possible to redesign proteins efficiently by simulating the natural evolutionary mutation, selection, and amplification in the lab.
"Protein engineering is the process of developing useful or valuable proteins. It is a young discipline, with much research taking place into the understanding of protein folding and recognition for protein design principles." (Source- Wikipedia)
With the help of protein engineering, researchers modify a protein sequence by substituting, inserting, or deleting nucleotides in the encoding gene to get a modified protein better suited for a particular use or purpose than the unmodified protein.
AI in Protein engineering enhances protein properties like stability and functioning to get modified proteins. Researchers have provided machine learning approaches in several studies that expedite the protein engineering process. Given that AI-based protein structure prediction models utilize to test a significant number of unique sequences virtually, there has recently been an increased interest in applying machine learning to support protein redesign.
Pharmaceutical research and development now place a high priority on protein redesign and engineering. There are countless potential mutations for each protein. It is not feasible to synthesize every sequence or look at every variant with potential functional applications.
"Deep learning is part of a broader family of machine learning methods based on artificial neural networks with representation learning." (Source- Wikipedia)
Deep learning algorithms help in protein engineering by easing the experimental burden of techniques like directed evolution, which includes numerous rounds of mutagenesis and high-throughput screening. After being trained on protein sequence databases, they simulate and forecast the fitness of every potential target protein sequence.
As the volume and diversity of protein sequences in public databases continue to increase rapidly, the challenge of accurately predicting function for highly divergent sequences becomes increasingly demanding.
Here is where deep learning in protein engineering comes into the picture. It enhances the quality of protein engineering and reduces the cost. There are countless mutations of protein that Deep Learning can develop.
Several evaluation metrics assess the AI-based protein structure predictions and measures on a wide range of public and private data sets. The Convolution Neural Network created using the structural data of the proteins and NLP models on protein sequence are most relevant for protein redesign.
Deep Learning-guided protein engineering represents a novel approach to optimizing complicated protein functionalities. Deep-learning algorithms predict protein function using data rather than a thorough model of the underlying physics or biological pathways. They speed up protein engineering by extracting information from all measured variants and using it to identify variants that are likely to be improved.
This review explains how to gather protein data, train machine-learning models, and use trained models to drive engineering. We provide recommendations at each level and consider future machine learning potential to enable the identification of new protein functions and identify the relationship between protein sequence and function.
Deep learning in protein engineering predicts the three-dimensional structure of proteins to design new proteins or improve existing proteins' performance. Deep Learning algorithms can learn to make predictions from data. It is a powerful tool that solves several problems in protein engineering.
For example, AlphaFold is a deep learning-based algorithm for accurate protein structure prediction. AlphaFold incorporates physical and biological knowledge about protein structure, leveraging multi-sequence alignments, into the design of the deep learning algorithm. By understanding the three-dimensional structure of proteins, scientists can develop drugs that bind to specific proteins and modulate their activity.
The innovative field of Deep Learning in protein engineering influences the creation of new proteins. It can use pre-trained networks with self-supervised training to understand the characteristics of "protein-like" sequences. To create strings with the desired property, one must first solve a complex problem: developing a reliable visionary for this attribute. Typically, one needs to define the design, the loss function, and a training set. Generate (potentially several) lines with the desired quality. Finally, experimental validation still needs to determine whether these created sequences have the necessary feature.
Deep learning-guided protein engineering represents a novel approach to optimizing complicated protein functionalities. Deep-learning algorithms predict protein functions using data rather than a model of the underlying physics or biological pathways. They speed up protein engineering by extracting information from all measured variants and using it to identify variants that are likely to be improved.
Deep Learning in protein engineering provides recommendations at each level and considers future machine learning potential to enable the identification of new protein functions and identify the relationship between protein sequence and function.
Deep learning in Protein Engineering helps design new proteins or predict existing proteins' properties. Deep Learning allows us to create models that can learn from data and predict protein sequences.
There are many benefits to using deep learning in protein engineering. Deep Learning can help us better understand proteins' function and how they interact with other molecules. It helps us to develop and study different mutations of protein faster. Deep learning gives us cost and time-effective techniques to analyze large amounts of possible protein variations. Ultimately, Deep Learning will allow us to create better protein for various applications.
One challenge for AI in protein engineering is the limited data available for training deep learning models. Interpreting data from experiments can be time-consuming and expensive. Another challenge is the need to understand how deep learning models work.
For example, Proteins are often highly variable in their structure and function, making it challenging to design a Deep Learning model that can accurately learn from data.
Additionally, Deep Learning models often require large amounts of data to train, and protein data can be difficult and expensive. The results these models generate are verified and studied. Finally, protein engineering is relatively new, and there is still much to learn about how to use deep learning in this context. Despite these challenges, deep learning is still a promising tool for protein engineering, and we are constantly working to overcome these challenges.
There is no doubt that Deep Learning has revolutionized protein engineering. In the past, protein engineers would have to design and test new proteins by trial and error laboriously. But with Deep Learning, they can now use algorithms to automatically design proteins that are far more efficient and effective than anything created before.
So, what does the future hold for deep learning in protein engineering? The possibilities are endless. We may see even more amazing proteins designed and created, and Deep Learning may help us understand the mysteries of protein folding.
The potential for deep learning in protein engineering is vast. By harnessing the power of artificial intelligence, you can accelerate the designing and testing of new proteins, harnessing the power of Artificial intelligence to breakthroughs in treating diseases, developing new materials, and producing more efficient and sustainable fuels.
Deep learning is still in its early stages, but the potential for its applications in protein engineering is immense. As we continue exploring this technology's possibilities, you may soon find yourself on the cusp of a new era in protein engineering.
In recent years, AI in protein engineering has utilized deep learning algorithms to help design and optimize proteins. This technology is improving the efficiency of AI in protein engineering and helping to create new, better proteins. The field has made great strides in using deep learning to accelerate protein engineering. This blog provides an overview of how AI in protein engineering utilizes Deep Learning algorithms. If you found this blog informative, please share this content with your network and help us reach better. Visit our page for AI and Deep learning solutions to learn more.
You can subscribe to our blog to learn more about AI and Deep Learning in the protein engineering industry.